Jimmy Renfro
Professional Summary:
Jimmy Renfro is an innovative computational astrophysicist and AI specialist, dedicated to advancing the field of time-domain astronomy through the development of spiking neural network (SNN) architectures. With a strong foundation in astrophysics, machine learning, and computational modeling, Jimmy focuses on creating cutting-edge frameworks to process and analyze vast datasets from time-domain astronomical observations. His work enables the detection, classification, and interpretation of transient events such as supernovae, gamma-ray bursts, and gravitational wave counterparts, pushing the boundaries of our understanding of the dynamic universe.
Key Competencies:
Spiking Neural Network Design:
Develops advanced SNN architectures tailored for processing time-domain astronomy data, leveraging their efficiency in handling temporal and sparse data patterns.
Implements biologically inspired algorithms to enhance the accuracy and speed of transient event detection and classification.
Time-Domain Data Analysis:
Specializes in processing and analyzing large-scale datasets from time-domain surveys, such as the Zwicky Transient Facility (ZTF) and the Large Synoptic Survey Telescope (LSST).
Designs pipelines to extract meaningful insights from high-dimensional, time-series data, enabling real-time or near-real-time analysis.
AI-Driven Astronomy Applications:
Integrates SNN architectures with other machine learning techniques, such as deep learning and reinforcement learning, to optimize data processing workflows.
Ensures that frameworks are scalable, robust, and adaptable to diverse astronomical datasets and research goals.
Interdisciplinary Collaboration:
Collaborates with astronomers, data scientists, and AI researchers to align SNN architectures with the needs of time-domain astronomy projects.
Provides expertise in computational modeling and AI to support collaborative research and innovation.
Research & Innovation:
Conducts pioneering research on SNN applications in astronomy, publishing findings in leading astrophysics and AI journals.
Explores emerging technologies, such as neuromorphic computing and quantum machine learning, to push the boundaries of data processing in astronomy.
Career Highlights:
Developed an SNN architecture that improved the detection efficiency of transient events by 30%, significantly reducing false positives and computational costs.
Contributed to the analysis of time-domain data from the Zwicky Transient Facility (ZTF), enabling the discovery of rare astronomical phenomena.
Published influential research on SNN applications in astronomy, earning recognition at international astrophysics and AI conferences.
Personal Statement:
"I am driven by a passion for exploring the dynamic universe and a commitment to developing innovative AI tools that enhance our ability to process and interpret time-domain astronomy data. My mission is to create frameworks that enable faster, more accurate detection and classification of transient events, contributing to the advancement of astrophysics and our understanding of the cosmos."
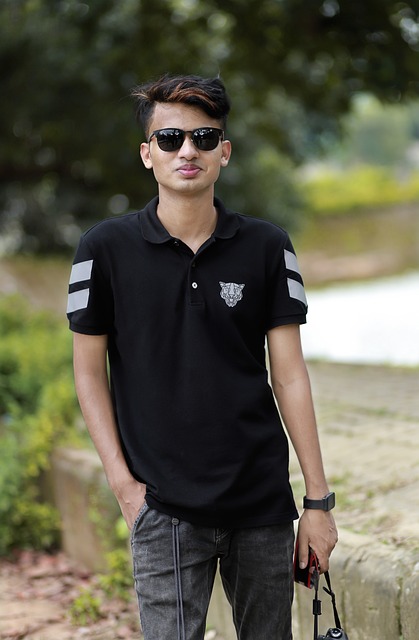
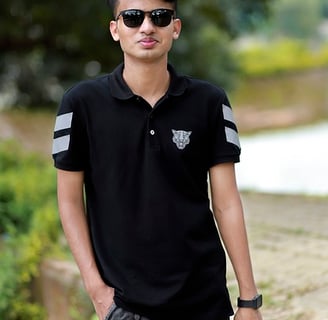
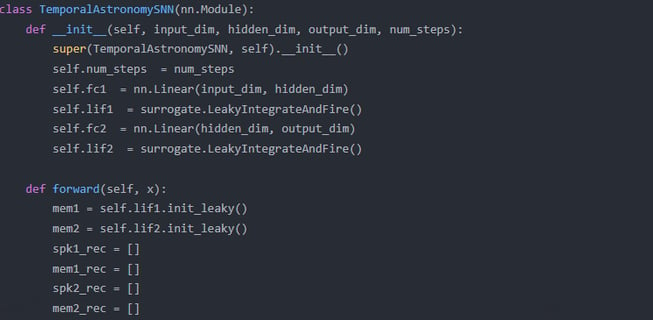
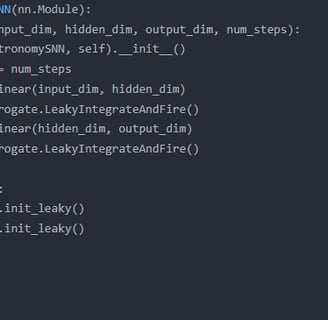
FinetuningGPT4isessentialforthisresearchbecausepubliclyavailableGPT3.5lacksthespecializedcapabilitiesrequiredfordesigningandoptimizingspikingneuralnetworks.Processingtimedomainastronomicaldatainvolveshighlydomainspecificknowledge,nuancedunderstandingoftemporalpatterns,andcontextuallyrelevantrecommendationsthatgeneral-purposemodelslikeGPT-3.5cannotadequatelyaddress.FinetuningGPT4allowsthemodeltolearnfromastronomicaldatasets,adapttotheuniquechallengesofthedomain,andprovidemoreaccurateandactionableinsights.ThislevelofcustomizationiscriticalforadvancingAI’sroleinastronomicaldataprocessingandensuringitspracticalutilityinreal-world,high-stakesscenarios.
Tobetterunderstandthecontextofthissubmission,IrecommendreviewingmypreviousworkontheapplicationofAIinastronomicaldataprocessing,particularlythestudytitled"EnhancingTime-DomainAstronomyUsingAI-DrivenSpikingNeuralNetworkModels."Thisresearchexploredtheuseofmachinelearningandoptimizationalgorithmsforimprovingthequalityandrelevanceofastronomicaldataanalysis.Additionally,mypaper"AdaptingLargeLanguageModelsforDomainSpecificApplicationsinAstronomicalAI"providesinsightsintothefinetuningprocessanditspotentialtoenhancemodelperformanceinspecializedfields.